IoT is digitizing the world by embedding billions of sensors in almost everything today. A large amount of data generated by these sensors get collected and analyzed using AI-enabled IoT solutions. Moreover, with the increasing adoption of technologies like artificial intelligence (AI), deep learning and big data analytics, it becomes important to process large amounts of data efficiently through edge computing in real-time. The networking, storage and computing competencies are now available at the edge, but its speed and capacity to process data will improve only with time. Advancements like Non-Volatile Memory Express (NVMe) will enhance the performance and adoption of AI at the edge.
Cloud computing will always remain an important part of the IoT systems for data processing. However, edge computing is a faster method to enable real-time decision-making for many applications thereby providing computing and analytics abilities to end devices.
Advantages of AI-enabled decision-making at the edge:
Reduced latency
With AI at the edge, devices process data faster than the centralized IoT models and have faster response times i.e. reduced latency. This allows for real-time processes like data creation, decisions and actions.
Greater security
AI capabilities at the edge reduce the data processing time to less than a few milliseconds. This eliminates the risk of data tampering during the data transfer. The devices are incorporated with enhanced security features for greater security.
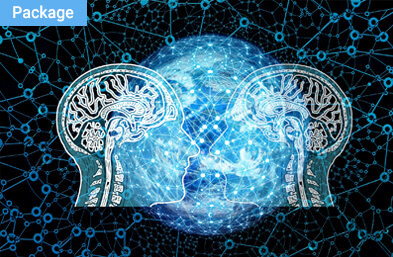
AI strategy and planning workshop
Artificial intelligence is transformational, and adopting this technology will enable companies to reshape their strategic vision so that they no longer rely on what they think is true, rather, leverage factual-based decision-making capabilities.
Greater customer experiences
AI at the edge gives insights in real-time, which helps in addressing customer concerns efficiently and to enhance customer service.
Cost effectiveness
Company owners are always concerned about the costs linked with the adoption of IoT solutions. Edge computing enables them to select suitable architecture networks like bandwidth and computation power to gain more with less expenses as compared to the cloud. AI at the edge will reduce costs for data communication as less data will be transmitted to the cloud.
Reliability and security
Edge computing saves the data from traveling to the cloud by storing and processing it locally and making sure that no data is lost and no operational failures are faced. This way you can avoid the problems like streaming and storing a lot of data to the cloud that may make your system vulnerable.
According to a study from IDC, 45 % of all data created by IoT devices will be stored, processed, analyzed and acted upon close to or at the edge of a network by 2020.
Significance of AI at the edge
IoT devices generate a huge amount of data in an industrial setup, and it is difficult to transfer this data to the cloud effectively and economically. At present, not even 1% of the generated data is analyzed by the centralized cloud. The way IoT devices are thriving, the amount of data that they generate will grow with time. In such a scenario, central servers will not have the capacity to process all the data efficiently and effectively. This means that the cloud AI model will not be effective in many situations.
AI at the edge, processes and analyzes data at the edge or at end-devices that are close to the location of the data source. Hence, less data is transferred to the central cloud. The fact that data is not stored centrally will ease data privacy concerns. It also improves the performance time since data doesn’t have to travel amid hub and edge.
Some use cases showcasing the benefits of AI at the edge:
Self-driving cars
Every self-driving car needs edge computing as it must make real-time decisions based on the data that it collects in real-time. And since these decisions must be taken instantly, there is not enough time to send the data to the cloud for processing. Hence the data needs to be processed at the edge.
Airplane monitoring
Modern aircrafts deploy thousands of sensors that generate a huge amount of data. This data can be processed immediately at the edge to make certain decisions for corrections in the flight to ensure safety.
Manufacturing
The incorporation of IoT and AI in industrial equipment helps manufacturers to collect and analyze data for extraction of useful insights. This helps in predictive maintenance, improving energy efficiency, minimizing costs and increasing product uptime. Companies can also customize production to meet consumer requirements.
Smart cities
Many cities are now having abundant traffic sensors, video surveillance cameras and other monitoring devices. These sensors constantly collect data at multiple locations and AI at the edge analyzes it in real-time to make timely decisions to keep traffic moving and ensuring the safety of residents.
Conclusion
It is true that most of the AI and ML technologies are still contained in giant and strong cloud servers, but this may not stay the same always. AI at the edge enables the devices on the network edge to utilize learning models, data processing and analytics from the same location where the data is collected. And in the coming years, artificial intelligence is expected to become a norm for the workplace. AI-powered IoT systems will create big differences in the realization of smart homes, offices, factories, cities and more. To know more about the benefits of AI at the edge, talk to our experts.